The Ultimate Guide to Using an Image Labelling Tool for Business Success
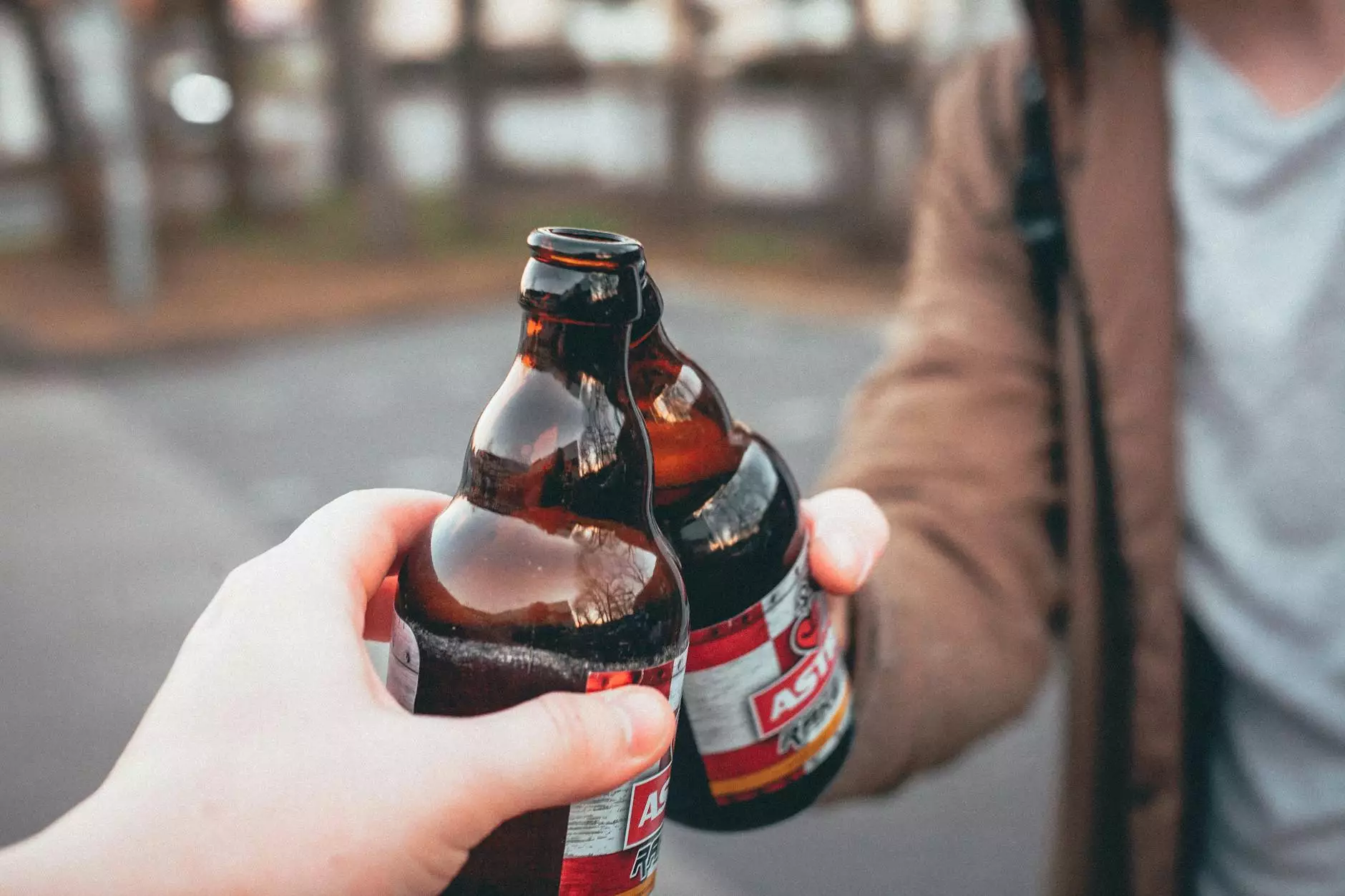
Introduction to Image Labelling Tools
In today’s rapidly evolving digital landscape, businesses are increasingly turning to artificial intelligence (AI) and machine learning (ML) to create a competitive edge. Central to the success of these technologies is the availability of high-quality, accurately labelled data. This is where an image labelling tool becomes indispensable.
What is an Image Labelling Tool?
An image labelling tool is software that facilitates the process of annotating images with labels that help machines in understanding visual content. These tools are essential for training computer vision algorithms, which are used in a variety of applications, from autonomous vehicles to facial recognition systems.
The Importance of Data Annotation
Data annotation is critical because it directly affects the output of machine learning models. The quality of the input data determines the efficiency and accuracy of the model’s predictions. Without precise annotations, models can misinterpret images, leading to poor performance and unanticipated results. Therefore, choosing the right image labelling tool is key for any business leveraging these technologies.
Key Features to Look for in an Image Labelling Tool
When selecting an image labelling tool, there are several features that can significantly impact your data annotation process:
- User-Friendly Interface: A straightforward, intuitive layout enables faster onboarding and less training for new users.
- Collaboration Capabilities: Multi-user functionality allows teams to work concurrently, enhancing productivity.
- Customizable Annotation Options: The ability to tailor labels and drawing tools according to specific needs fosters accuracy.
- Quality Control Features: Built-in review systems ensure the labelled data meets predefined standards.
- Integration with Other Tools: Compatibility with data management systems and machine learning platforms can streamline workflows.
- Scalability: The tool should effectively handle large datasets, accommodating the growth of your business.
Advantages of Using an Image Labelling Tool for Businesses
Incorporating an image labelling tool into your data preparation process can yield several advantages:
Enhanced Efficiency
Manual image annotation is often time-consuming and prone to human errors. An image labelling tool automates many of these processes, enabling businesses to achieve higher throughput in data processing.
Improved Accuracy
These tools often come equipped with advanced algorithms, or even AI capabilities, that enhance the precision of annotations. This leads to better-trained models, resulting in improved decision-making.
Cost-Effectiveness
By reducing the time and resources needed for data annotation, businesses can save significantly. Fewer resources allocated to data preparation allows for a reallocation of funds towards core business development initiatives.
Better Collaboration and Project Management
With collaborative features, teams can coordinate more effectively, share insights, and manage annotation projects seamlessly, enhancing overall project management.
How to Effectively Use an Image Labelling Tool
While choosing an image labelling tool is important, understanding how to leverage it effectively is equally crucial. Here are some strategies:
1. Define Your Objectives
Before diving into the annotation process, clearly outline the objectives of your project. Understanding the specific outcomes required will help guide your annotation process.
2. Select the Right Tool
Based on your objectives, select an image labelling tool that aligns with your needs. Ensure it has the necessary features and can scale with your project demands.
3. Train Your Team
A well-trained team can significantly improve the quality of annotations. Conduct training sessions to familiarize team members with both the software and the labelling guidelines.
4. Regularly Review and Validate Annotations
Implement a review system where annotations are periodically checked for accuracy. This helps in maintaining a high standard of data quality which is crucial for effective machine learning.
5. Keep Data Security in Mind
Ensure that the image labelling tool you choose complies with data protection standards to safeguard sensitive information.
Case Studies: Successful Implementation of Image Labelling Tools
To illustrate the effectiveness of image labelling tools, let’s explore some success stories from various industries:
Case Study 1: Healthcare Industry
In the healthcare sector, a hospital used an image labelling tool to annotate images from patient scans to develop a diagnostic model for detecting tumors. By employing a robust data annotation platform, they achieved an accuracy improvement of over 30% in their diagnoses, significantly enhancing patient outcomes.
Case Study 2: Autonomous Vehicles
A leading automotive company integrated an image labelling tool to annotate thousands of images from their sensors for self-driving technology. This process enabled the AI to learn how to identify road signs, pedestrians, and obstacles, leading to safer driving conditions and better vehicle functionality.
Case Study 3: Retail Industry
A prominent retail chain utilized an image labelling tool to analyze customer interactions through images taken in-stores. By annotating these images, they gained insights into customer behavior and preferences, aiding marketing strategies and inventory management.
The Future of Image Labelling Tools
The landscape of image labelling tools is continually evolving, driven by advancements in AI and machine learning. The integration of machine learning algorithms into annotation processes allows for more sophisticated capabilities such as:
- Automated Annotations: Future tools may leverage AI to provide suggestions or even automatically label images, reducing manual input.
- Real-Time Collaboration: As remote work becomes more common, tools will likely evolve to enable real-time collaboration across global teams seamlessly.
- Enhanced Security Protocols: With increasing concerns about data privacy, the future will see improved security measures to protect sensitive information.
- Greater Integration with AI Workflows: As AI systems advance, image labelling tools will become more integrated into end-to-end AI workflows, streamlining data management.
Conclusion
The right image labelling tool is essential for businesses looking to harness the power of AI and machine learning effectively. By improving the quality and efficiency of data annotation, organizations can unlock new opportunities, enhance performance, and remain competitive in a data-driven world. Investing in a top-notch data annotation tool, such as the offerings from Keylabs.ai, can set your business on the path to success.