The Essential Guide to Image Annotation Tool for Machine Learning
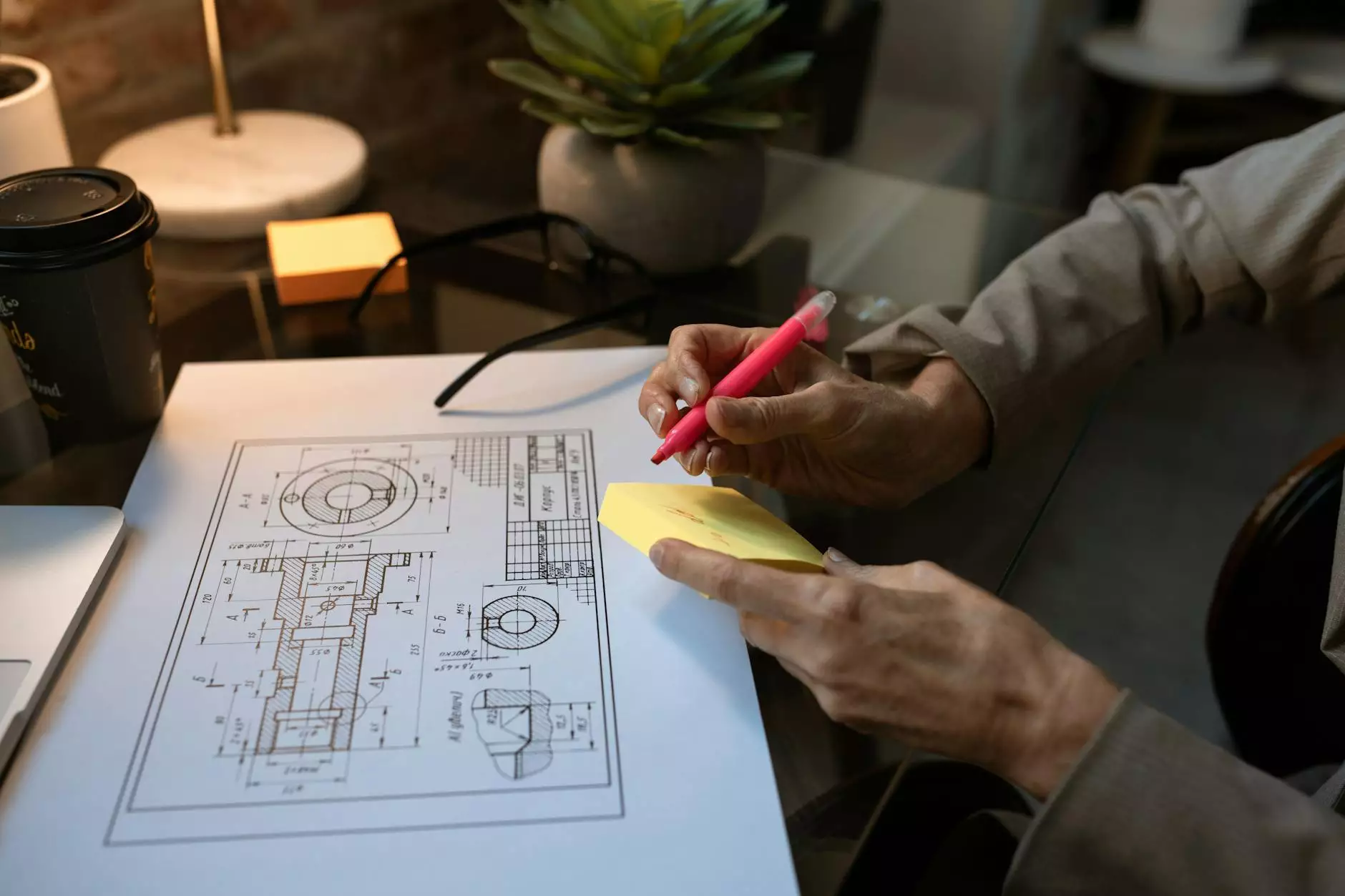
In the rapidly evolving world of artificial intelligence (AI) and machine learning (ML), image annotation tools for machine learning have emerged as pivotal instruments. They facilitate the accurate labeling of image data, which is essential for training robust machine learning models. This article offers an in-depth exploration of image annotation tools, their significance in data annotation platforms, and the benefits they bring to businesses looking to leverage machine learning.
Understanding Image Annotation
Image annotation is the process of labeling images with corresponding tags or information that signify elements within the image. The primary goal of this task is to prepare data for machine learning algorithms, enabling these models to understand and interpret the visual world. Image annotation tools for machine learning offer a range of functionalities that make this process efficient and effective.
The Role of Image Annotation in Machine Learning
At the core of every successful machine learning project lies a well-annotated dataset. Here’s why image annotation is critical:
- Data Quality: High-quality annotations lead to better-trained models.
- Feature Recognition: Annotated images help ML algorithms learn specific features, like faces, objects, and landscapes.
- Improvements in Accuracy: Comprehensive image data with accurate labels results in improved accuracy and performance of machine learning models.
Types of Image Annotation Techniques
There are several techniques used in image annotation, each suited for different use cases. Understanding these techniques can significantly enhance your ability to select the appropriate image annotation tool for machine learning.
1. Bounding Box Annotation
Bounding box annotation involves drawing boxes around objects of interest within an image. This technique is particularly useful in object detection tasks where identifying the location of an object is crucial.
2. Semantic Segmentation
Semantic segmentation goes a step further by classifying each pixel within the image. This method is ideal for applications that require an understanding of the context of the scene, such as autonomous driving where it's important to identify every element in the environment.
3. Polygon Annotation
Polygon annotation is favored for complex shapes where bounding boxes would be inadequate. By outlining the shape of an object with multiple points, this method provides a detailed analysis that enhances model training.
4. Landmark Annotation
In landmark annotation, specific points (landmarks) are marked on an image, often used for facial recognition systems. This technique greatly aids in training models that focus on specific features like eyes, nose, or mouth.
How to Choose the Right Image Annotation Tool
With numerous options available, selecting the right image annotation tool can be daunting. Here are some key considerations for businesses:
- Ease of Use: The tool should have a user-friendly interface that allows team members to annotate images efficiently.
- Integration Capabilities: Ensure that the tool can seamlessly integrate with your existing workflows and technology stack.
- Scalability: As your data grows, the annotation tool should be able to scale accordingly, handling increased volumes without compromising performance.
- Types of Annotations Supported: Different projects require different annotation techniques; choose a tool that supports a wide range of them.
- Collaboration Features: A good tool should promote collaboration among team members, allowing real-time feedback and sharing of insights.
- Cost-Effectiveness: Analyze your budget and the overall value the tool brings to your project to ensure a good return on investment.
The Benefits of Utilizing Image Annotation Tools
Implementing a reliable image annotation tool for machine learning can bring numerous benefits to your organization. These include:
1. Enhanced Data Quality
With precise annotations, the quality of the data used for training machine learning models is significantly improved. High-quality data is crucial for achieving desirable outcomes in machine learning applications.
2. Improved Efficiency
Automated image annotation tools can speed up the process, allowing businesses to scale quickly without the overhead of manual labeling.
3. Skilled Workforce Utilization
By automating repetitive tasks, your highly skilled team can focus on more strategic tasks that can add value to your business.
4. Flexibility and Customization
Many image annotation tools offer customization options, allowing businesses to adapt the tool to meet specific project needs and workflow requirements.
Case Studies: Successful Implementation of Image Annotation Tools
Several companies worldwide have harnessed image annotation tools to propel their machine learning efforts. Here are a few case studies highlighting successful implementations:
Case Study 1: Autonomous Vehicle Technology
A leading automotive company employed a sophisticated image annotation tool to handle the vast amounts of data collected by its fleet of autonomous vehicles. By using polygon annotation and semantic segmentation, they were able to train their vehicles to recognize road signs, pedestrians, and other vehicles in real time.
Case Study 2: Healthcare Imaging Analysis
A healthcare company utilized an image annotation platform for analyzing medical images to detect anomalies. Utilizing landmark annotation techniques, they significantly reduced the time taken for radiologists to diagnose conditions, improving patient outcomes and operational efficiency.
Case Study 3: E-commerce Visual Search
An e-commerce giant incorporated image annotation tools to enhance its visual search capabilities. By annotating millions of product images, they improved their algorithms to deliver better search results, greatly enhancing user experience and increasing sales conversion rates.
The Future of Image Annotation Tools
As the demand for machine learning continues to surge, the image annotation field is expected to evolve rapidly. Innovations such as AI-assisted annotation are on the horizon, potentially streamlining processes further while improving accuracy.
Businesses looking to stay ahead in the AI arena will benefit greatly from adopting state-of-the-art image annotation tools, remaining adaptable to emerging technologies and methodologies.
Conclusion
In conclusion, image annotation tools for machine learning are indispensable assets for organizations aiming to build effective machine learning models. They not only improve data quality and training efficiency but also empower businesses to make informed decisions based on comprehensive datasets. By investing in the right data annotation tools, companies can enhance their capabilities and stay competitive in an increasingly technology-driven landscape. For those looking for a reliable partner in this domain, KeyLabs.ai offers cutting-edge solutions tailored to meet diverse business needs in data annotation.